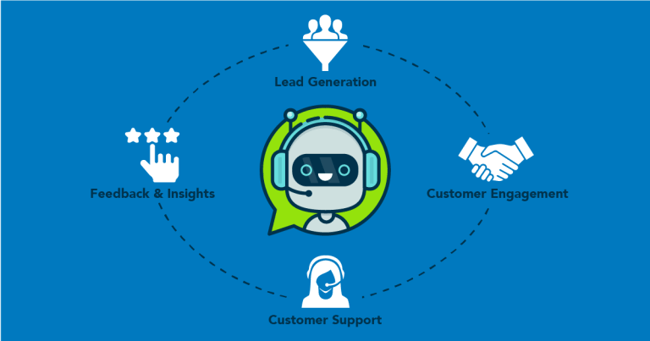
Conversational AI is everywhere, as both consumers and businesses are looking for ways to interact with technology more humanly. Conversational AI is the next step in the evolution of human-machine interactions, allowing you to build applications that can understand natural language and respond with appropriate responses based on context and user preference.
Natural language understanding (NLU)
Natural language understanding (NLU) is a subset of artificial intelligence that focuses on understanding human language. It is a combination of natural language processing (NLP), machine learning (ML), and deep learning. NLU is used in chatbots, voice assistants, and other conversational interfaces.
NLP focuses on parsing sentences to break them down into different parts of speech like nouns or verbs. ML has been around for a while now but only recently has it been able to accurately interpret human speech thanks to advancements in deep learning algorithms like neural networks.
The main function of NLU is understanding what users want without requiring them to explicitly state it within an app or website interface by using natural language processing (NLP). For example: if you were creating an app that helps people find restaurants near their current location then you would want your customers’ input to be understood no matter how they say something like “can you tell where there’s good Mexican food close by?”
Natural language generation (NLG)
Natural language generation (NLG) is a key feature of conversational AI. A chatbot can generate responses to user queries that are natural and conversational. NLG can be used to create a conversational experience for the user, or it can be used to create FAQs, tutorials, and other content in your app.
It’s important that NLG responses feel like they come from an actual person — not just any random response; otherwise, users will get frustrated with your chatbot and lose interest before you have a chance to show off its full potential.
Interactive learning
Interactive learning is a technique to improve the performance of pre-trained models. A typical use case for this method is when you have trained the model in advance with a small amount of data and now want it to be able to handle new content.
Interactive learning is done by having a human train the model, which means providing examples and getting feedback from them. This process can be repeated until your model reaches an acceptable level of accuracy across all different types of content that you might need it for.
Adaptability to user contextual information
Context is key in any conversation, especially if you want your AI to be conversational and engaging. This means that it needs to understand how its words will affect the user in context and adapt accordingly. For example, if a user asks for information about a product when they’re already logged into their account, then the AI should provide their detailed product information rather than asking them again what they want. The other thing is that contextual information can change over time so an AI should be able to update itself on these changes as well.
Conclusion
Conversational AI is the future of customer service. It’s already making a big impact in industries like healthcare and hospitality, with many more industries sure to follow. The technology is still being refined and developed but you can already see how useful it will be in helping humans interact with other people or machines more naturally through conversation.